ExoGAN concept
ExoGAN is made of two networks pitting one against the other during the training phase. They learn from a training set which can represent many different subjects. In our specific problem we trained a DCGAN on 10 million exoplanetary atmospheric transmission spectra generated by the TauREx retrieval code.
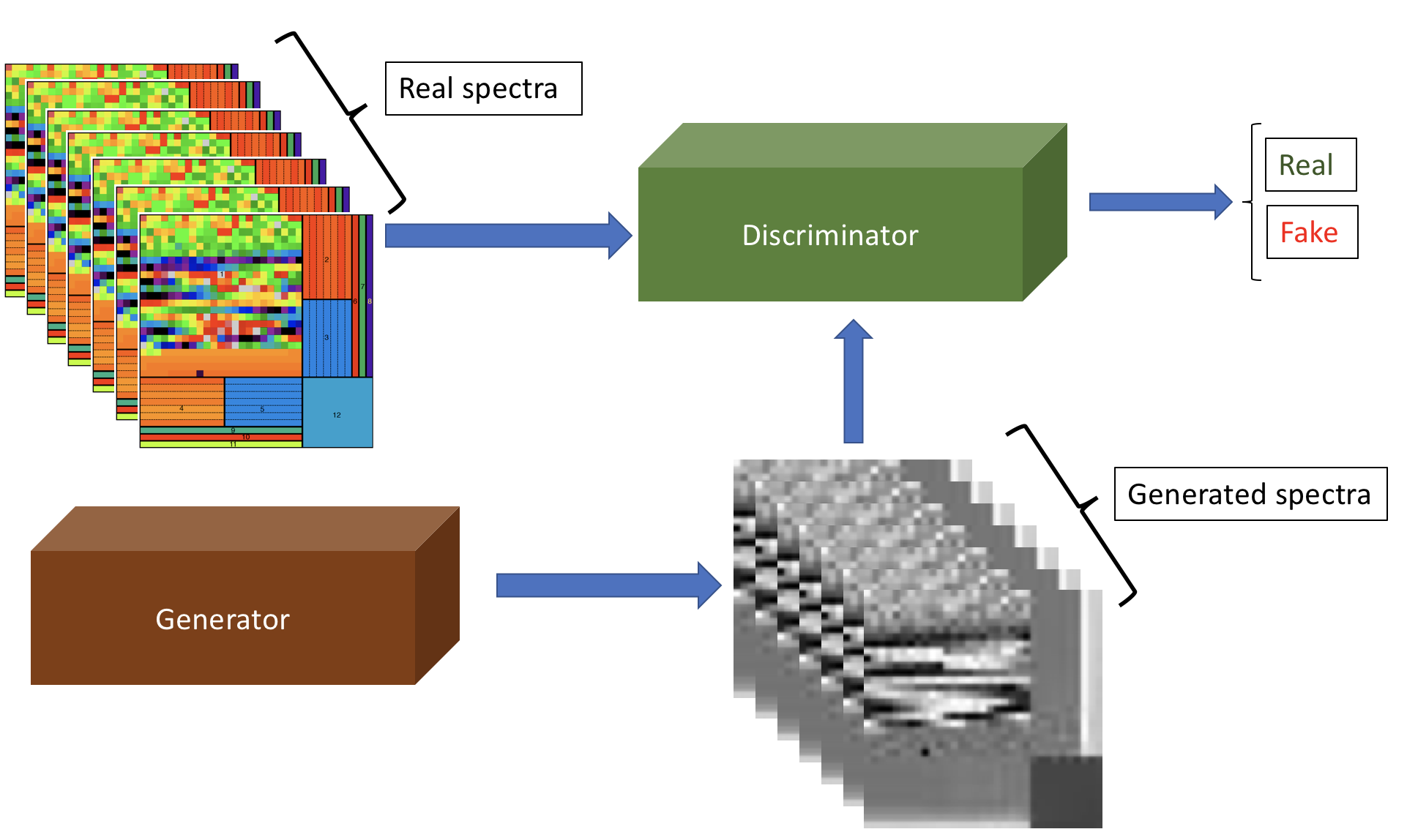
The transmission spectra and the parameters necessary to generate them are assembled into a unique QR-like code called ASPA (Atmospheric Spectral and Parameters Array), defining a fingerprint for a single exoplanetary atmosphere.
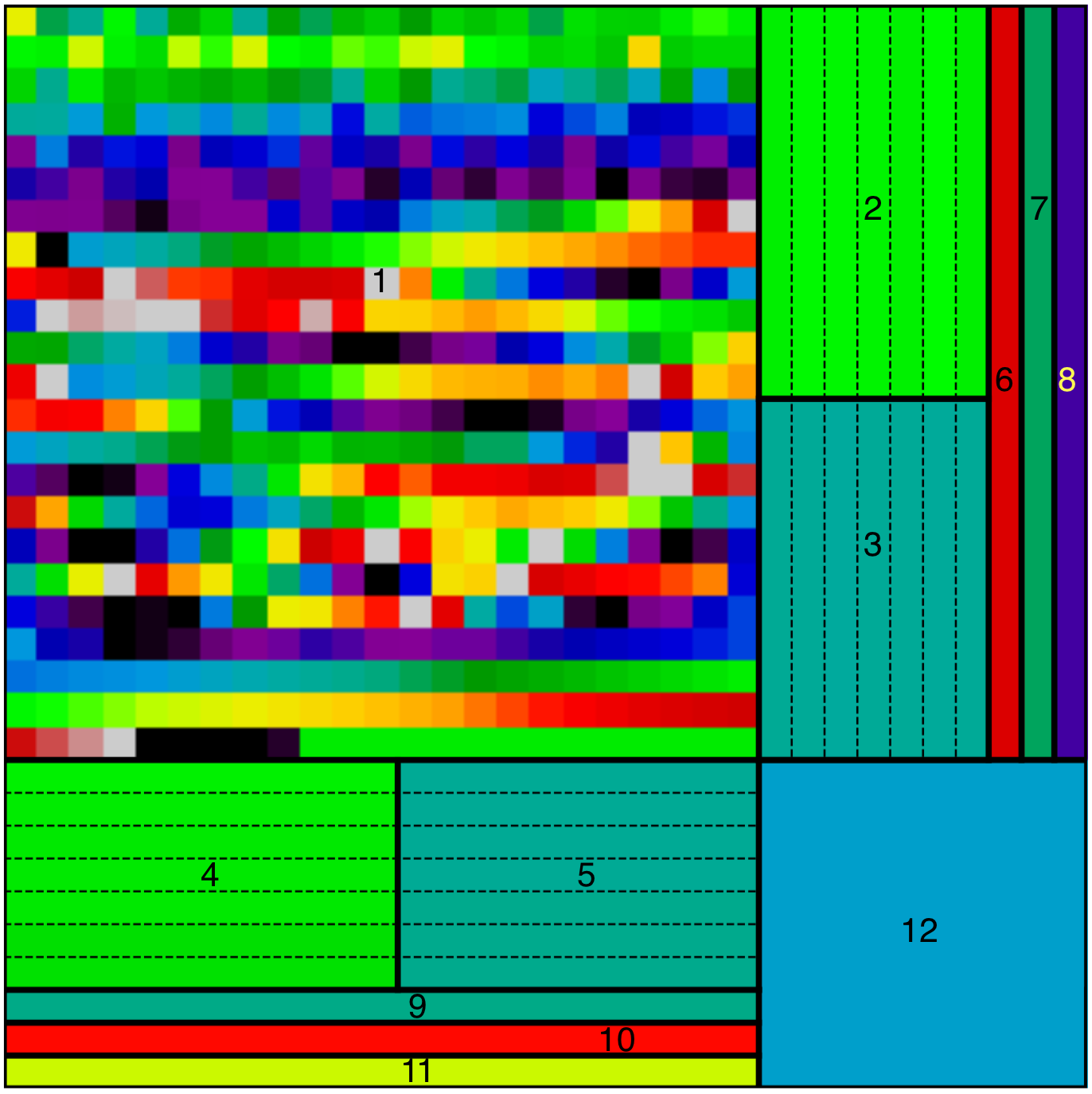
Using the inpainting technique introduced by Brandon Amos (http://bamos.github.io/2016/08/09/deep-completion) ExoGAN reconstructs both the parameters array and any missing part of the spectrum.
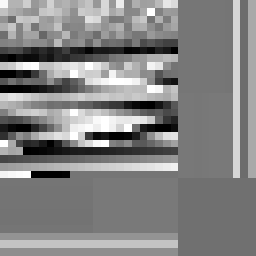
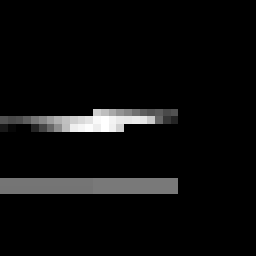
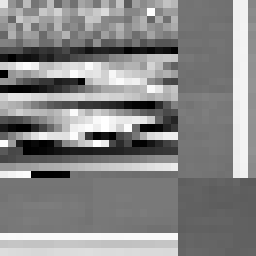
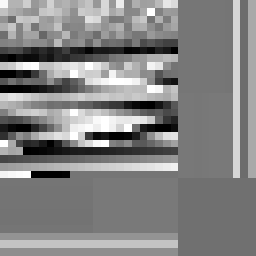
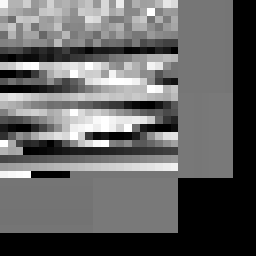
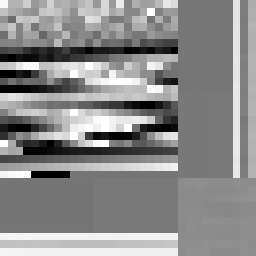
ExoGAN is able to analyse exoplanetary atmosphere and provides a very well constrained parameters space for atmospheric parameters. It returns results with an unprecedented speed endependently on the complexity of the atmospheric model we use. Deep Learning solution can offer us the possibility of including much more complex models in our atmospheric analysis and solve many computing-intensive tasks.